Artificial Intelligence and Autism: How Neural Networks Can Aid in Diagnosis.
Hello, friends!
In recent years, researchers have been increasingly exploring the potential of artificial intelligence (AI) in diagnosing autism spectrum disorders (ASD). Today, we’ll talk about a recent study by Spanish scientists, where they used three-dimensional convolutional neural networks (3D-CNN ResNet) to analyze body movements in children with autism
(Alberto Altozano, Maria Eleonora Minissi, Mariano Alcañiz, Javier Marin-Morales. Introducing 3DCNN ResNets for ASD Full-Body Kinematic Assessment: A Comparison with Hand-Crafted Features).
Why is this important?
Children with autism often exhibit unique motor characteristics: they may have unstable coordination, difficulties with movement planning, or unusual gestures. Researchers from the Polytechnic University of Valencia set out to determine whether ASD can be detected through body movements — and they did so using VR and neural networks.
How did the study work?
The study involved 39 children with ASD and 42 typically developing peers, all between the ages of 3 and 7. All children with ASD had previously been diagnosed using the ADOS-2 scale.
Participants were asked to complete 12 short tasks in virtual reality that involved object interaction and imitation. For example: throwing a ball, blowing bubbles, picking apples from a tree, or mimicking an avatar's movements.
Full-body motion (kinematics) was recorded — not just the hands, but also the torso, legs, and head. The recording was done using multiple systems installed in the room, so the children didn’t need to wear any sensors and could move and play freely.
After collecting the data, the researchers tested two approaches:
- Classical — where a specialist preselects which movements to analyze (e.g., speed or range of motion).
- Modern — where the neural network independently examines all the movements and identifies patterns without predefined features.
What were the results?
The 3D-CNN ResNet model achieved an accuracy of 85% based on just 1–3 minutes of movement data. It also demonstrated high reliability: AUC = 0.80, indicating good ability to distinguish ASD from typical development.
Unlike the manual approach, the neural network was less dependent on the specific task, meaning it could potentially be used in a variety of settings.
Why does this matter?
ASD diagnosis is often based on subjective behavioral assessment. This method offers an objective, digital alternative. It could be used for:
- screening programs,
- evaluating therapy progress,
- or even at home — if VR technologies become more accessible.
Are there any downsides?
Although neural networks proved to be a powerful tool, manual analysis still outperforms in certain specific tasks. Additionally, the technology requires high-quality equipment (VR, sensors) and time to train the models.
Conclusion
This study shows that body movements can serve as a reliable biomarker of autism, and artificial intelligence can be a powerful tool to detect those signals. In the near future, we might see affordable digital tests that help parents and professionals detect ASD faster and more accurately.
And while we wait for digital tests, we can read the full text of the article here.
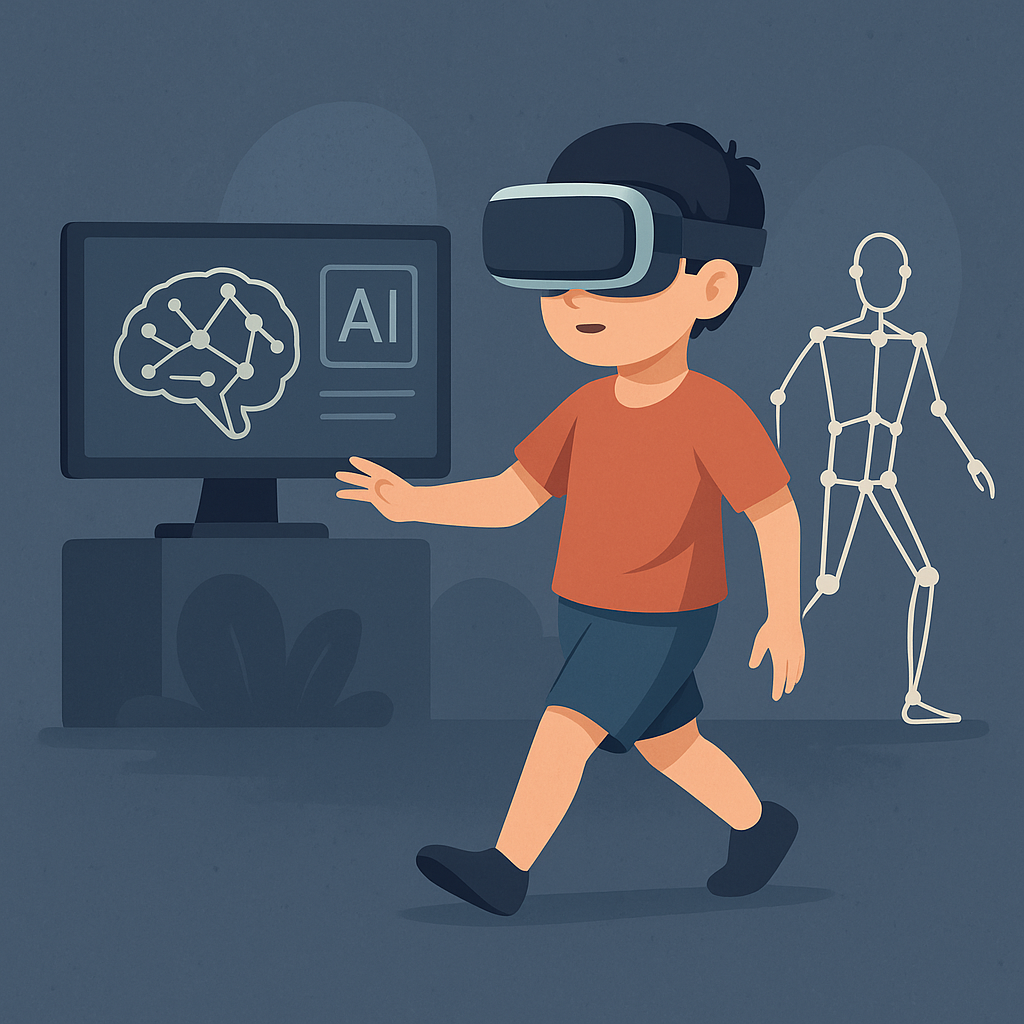